Unleash the Power of AI
Unlock the power of enterprise-ready AI and boost your entire software delivery lifecycle
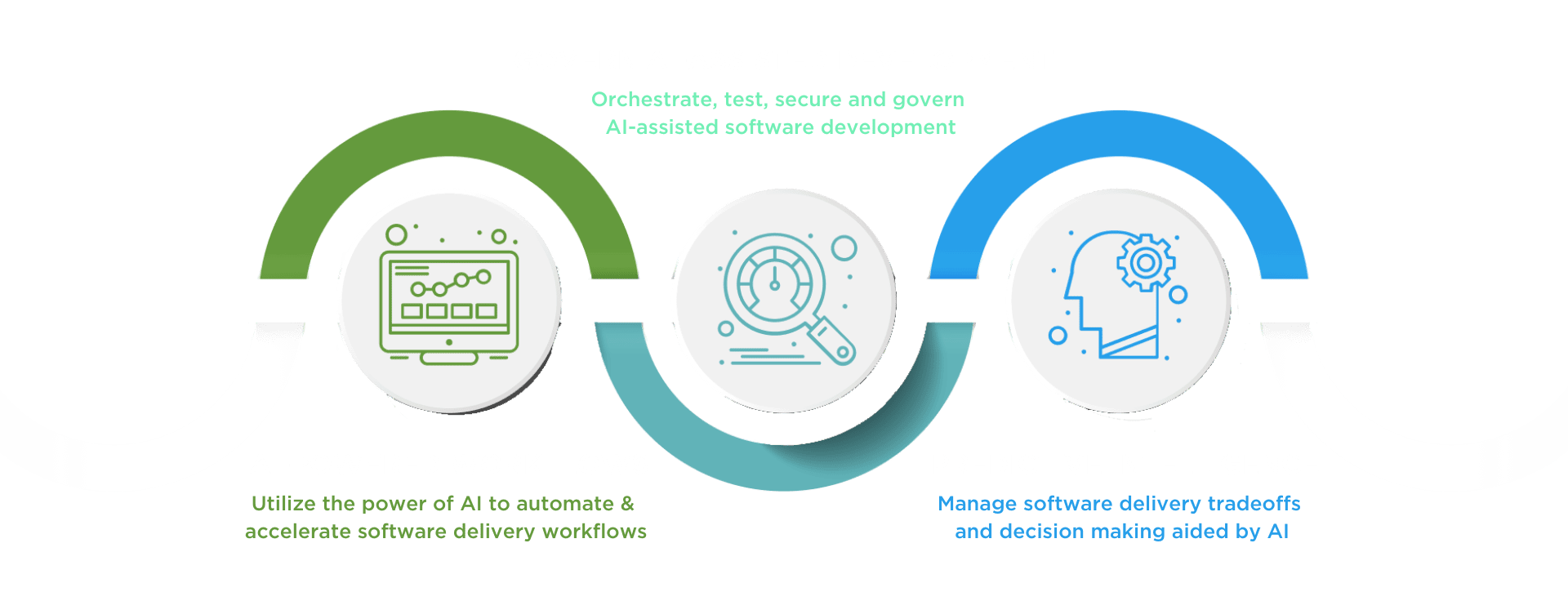
Our AI Capabilities
AI-POWERED WORKFLOWS
Test Creation
Simplify editing and creating test cases to increase quality and test coverage
Threat Insights
Gain more insights by analyzing threats and supplying changes to protected apps
Digital.ai Assistant
Identify information in planning and DevOps repositories without comprising privacy
GOVERN AI-ASSISTED DEVELOPMENT
Orchestrate & Govern AI-Based Software
Ensure checkpoints to avoid security vulnerabilities and reduce risk
Identify Higher Risks Code Changes
Absorb the increase in code created with scoring methods that identify code changes with a higher risk
Self-Service Onboarding Workflows
Optimize DevSecOps processes and increase developer productivity with self-guided, intelligent workflows
Establish Policies and Regulatory Control
Establish policies as code to ensure compliance and improve traceability
PREDICTIVE INTELLIGENCE
Flow Acceleration
Analyze release readiness and critical aspects of DevOps workflows to predict cycle time and increase deployment frequency
Quality Improvement
Derive valuable insights into preventing defect leakage through early detection and benchmarking code quality
Change Risk Prediction
Identify risk change, reduce change failures, and prepare immediate remediation for better MTTR
Service Management Process Optimization
Provide more predictive insights into IT operations by anticipating future service risks, mitigating risks of major incidents
"We are investing significantly in AI and machine learning across all domains of IT, and a good example of this commitment is our partnership with Digital.ai. By using Digital.ai's AI-driven Change Risk Prediction module we've been able to reduce business impact caused by change activity, increase the success of change releases and accelerate delivery of low-risk changes -- ultimately freeing our developers to be more productive.”
- CIO of Enterprise Infrastructure and Operations at one of the largest financial services companies in the US
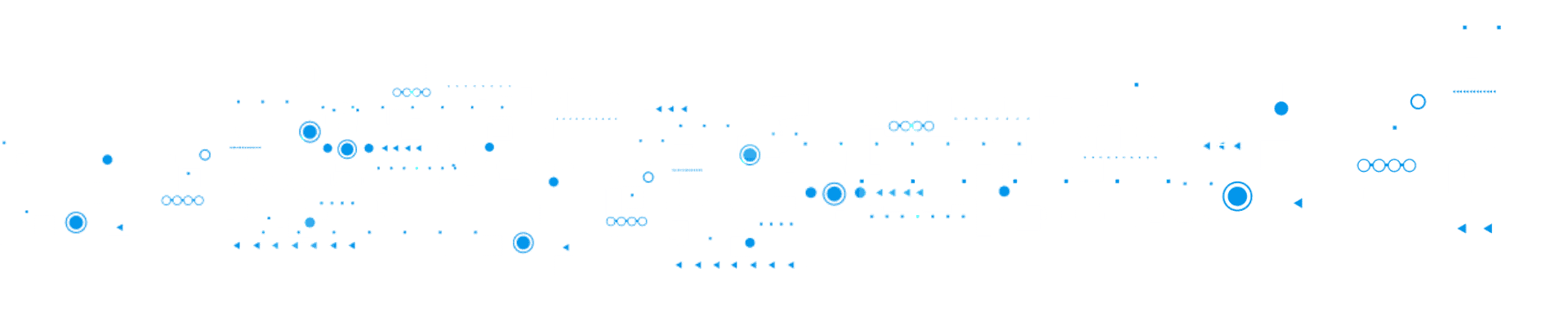
Related Resources
Read how Digital.ai is transforming the way development teams and organizations work by providing AI-capabilities designed to move traditional, static software delivery to automated processes and shift outdated reporting to dynamic models designed for how large scale, development organizations work today.
Unleash the Power of AI
Unlock the power of enterprise-ready AI and boost your entire software delivery lifecycle
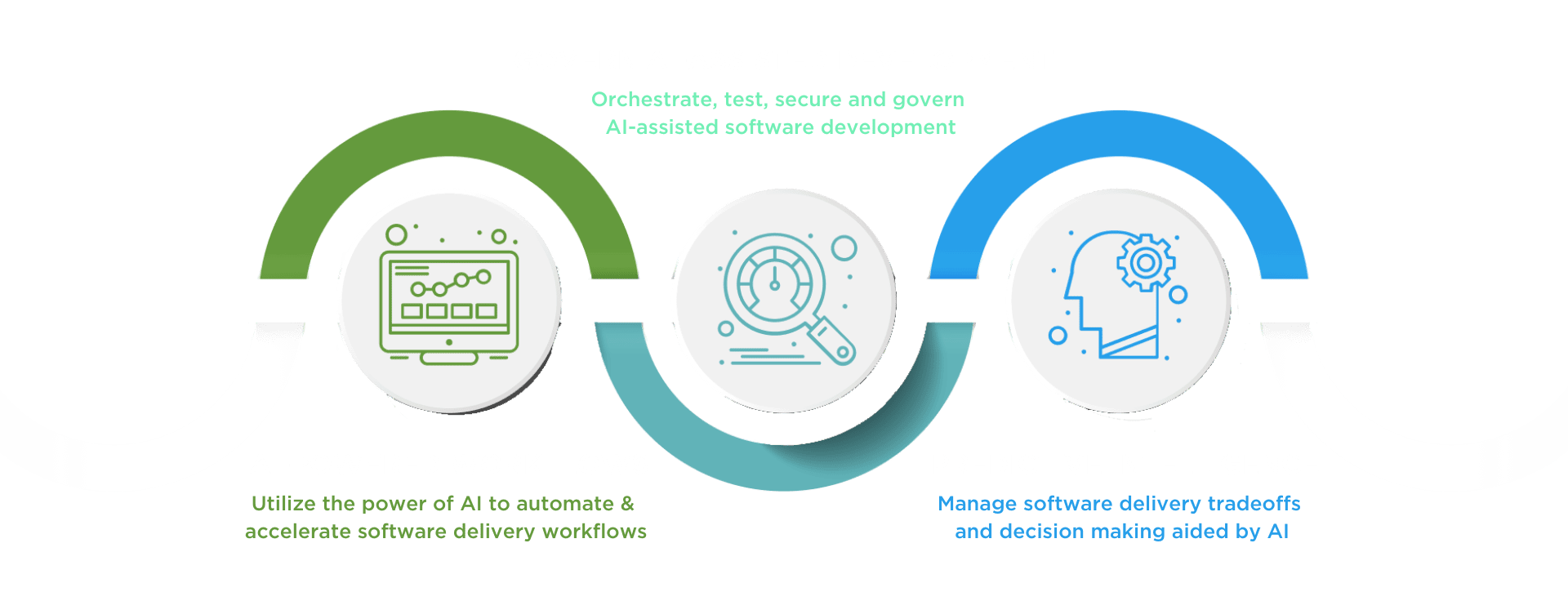
Our AI Capabilities
AI-POWERED WORKFLOWS
Test Creation
Simplify editing and creating test cases to increase quality and test coverage
Self Healing
Enhances test stability to reduce test maintenance
Threat Insights
Gain more insights by analyzing threats and supplying changes to protected apps
Digital.ai Assistant
Identify information in planning and DevOps repositories without comprising privacy
GOVERN AI-ASSISTED DEVELOPMENT
Orchestrate & Govern AI-Based Software
Ensure checkpoints to avoid security vulnerabilities and reduce risk
Identify Higher Risks Code Changes
Absorb the increase in code created with scoring methods that identify code changes with a higher risk
Self-Service Onboarding Workflows
Optimize DevSecOps processes and increase developer productivity with self-guided, intelligent workflows
Establish Policies and Regulatory Control
Establish policies as code to ensure compliance and improve traceability
PREDICTIVE INTELLIGENCE
Flow Acceleration
Analyze release readiness and critical aspects of DevOps workflows to predict cycle time and increase deployment frequency
Quality Improvement
Derive valuable insights into preventing defect leakage through early detection and benchmarking code quality
Change Risk Prediction
Identify risk change, reduce change failures, and prepare immediate remediation for better MTTR
Service Management Process Optimization
Provide more predictive insights into IT operations by anticipating future service risks, mitigating risks of major incidents
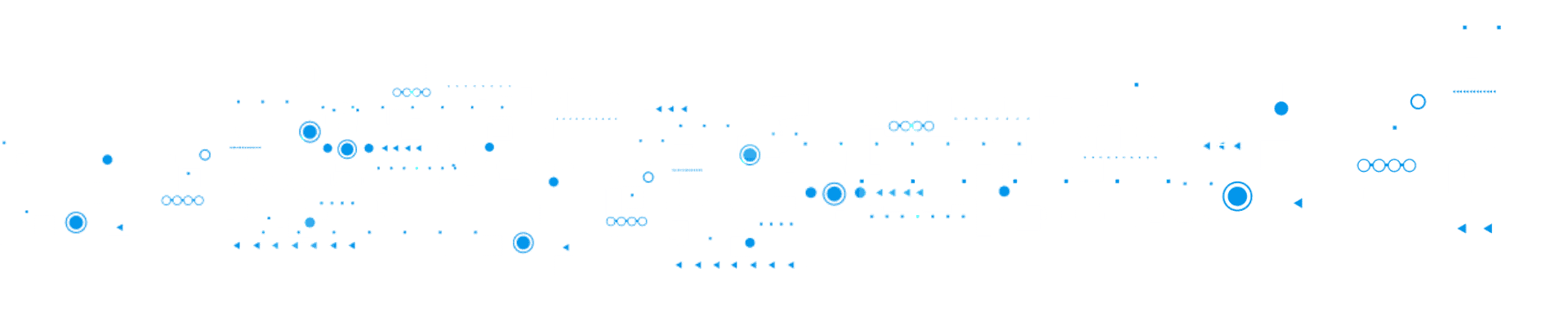
Related Resources
Read how Digital.ai is transforming the way development teams and organizations work by providing AI-capabilities designed to move traditional, static software delivery to automated processes and shift outdated reporting to dynamic models designed for how large scale, development organizations work today.
Want to learn more about AI from Digital.ai?
We can help you use AI to accelerate your digital transformation. Find out more today.
Our latest line of innovative products: some may not yet be available for purchase, some may be in limited supply, or some are generally available